Data & AI at Tesco with Venkat Raghavan, Director of Analytics and Science at Tesco
Venkat Raghavan is Director of Analytics and Science at Tesco. Venkat’s area of expertise is customer analytics, having been very heavily involved with the Tesco Clubcard loyalty program. Venkat also set up an analytics center of excellence to help break down data silos between teams. Previously, he was a Director of Analytics at Boston Consulting Group and Senior Director for Advanced Analytics & AI for Manthan and a Cross Industry Delivery Leader at Mu Sigma.
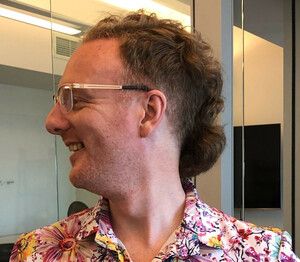
Richie helps individuals and organizations get better at using data and AI. He's been a data scientist since before it was called data science, and has written two books and created many DataCamp courses on the subject. He is a host of the DataFramed podcast, and runs DataCamp's webinar program.
Key Quotes
Now with the evolution of AI and GenAI, we're trying to build smart intelligence to connect all of this back to a product or a store or a category manager or a supplier. All of these are insights that get surfaced to our operators on a timely basis so that they can act on it, whether it's with the store manager or with the supplier or by changing the sort of the call patterns in our contact center
Whether you're a data engineering team or a data science team or any other team that you call yourself, the conversation should be about what are you committing to the board? What are the numbers that you're committing to? Because somewhere we have to understand that we are trying to enable the business to move forward. I think that's a little bit of a humble discussion that we need to have, saying ‘help us understand the commitment and therefore we can come back to you with the right ideas’. Now once you have the right ideas is when you need to set up the right governance and the right principles and the right controls with the engineering team. Saying, ‘look, we've got to work very tightly together’. The cadence of these governance meetings can be defined by the organization, but what's very important is to put the guardrails. Such as, there's a commitment to deliver so much growth in cash this year. Therefore, what we do with finance data and what we do with finance data science has to have a straight line between that number that has been committed to cash improvement. The fundamental principle is to go and understand commitments and try to align with commitments that are getting made as opposed to getting excited about initiatives and what's cool in the industry.
Key Takeaways
Utilize AI and data analytics to create personalized marketing and pricing strategies that cater to individual customer behaviors and preferences, enhancing customer engagement and loyalty.
Ensure that your data initiatives are directly tied to business objectives and KPIs, such as sales growth, margin improvement, and customer satisfaction, to clearly demonstrate their impact and value.
Implement predictive analytics to anticipate changes in costs, such as ingredient or commodity prices, enabling better negotiations with suppliers and more effective cost management.
Transcript
Richie Cotton: Hi Venkat, thank you for joining me on the show. So, just to begin with can you tell me the different areas that use data in Tesco?
Venkat Raghavan: Sure, uh, so before I get into data at Tesco, I'll probably talk about Tesco a little bit. Um, it's a European retailer, so for the global audience.
Tesco is a 100 plus year old company, which means that there's a rich heritage in terms of how we run retail. But what's more interesting is, Tesco has always been in the forefront of trying or innovating something with data. Just to give you a couple of examples, 30 years ago, 1995, Tesco was probably the first retailer to introduce a program called loyalty to the industry, which is called clubcard program, which meant that 30 years ago, there was a foresight that If we could get our customers to engage with us through the loyalty program, we could understand them better and we could personalize the experiences for them better.
I'm talking about 1995, much before a lot of the concepts were set. And the other example that I can talk about is, this was also the, one of the first retailers to get into food retail, pure food online retail. Uh, we all know that now it's a booming business. It's a, you know, food for now on, you know, food for tonight.
And all of that is a big concept right now. But again, 20 years ago, Tesco had the view that, uh, eventually food is going to become an online business. Um, and Tesco is probably the first business to reach 1 billion pounds in revenue for... See more
And pure food online. So the reason I wanted to share this is to say that Tesco, well it's a hundred year old company. It's a company that is constantly trying to innovate using data. Data led propositions. So with that backdrop, uh, what you now have is a business which is connected with uh, with customers through our loyalty program.
Which means that we get to understand what our customers think, feel, do with us in a very granular detail. We roughly have 223 million customers who are on Club Card. We have 16, 17 million active app users who use our apps, um, sort of interact with us. So there's a very rich understanding of customers in our business, uh, which basically leads to our marketing propositions, helps us in pricing, promotions.
A lot of how do we plan our trade and how do we compete with our competitors. But what's also very interesting is something that you don't see above the hood with Tesco is this tremendous amount of data science analytics that gets used in our finance. as an area and our people and colleague as an area and how we manage cost suppliers, how we run our supply chain.
I think, I think what really, you know, gets seen outside is all the cool things about customers and the experience that we create for customers. But what is the X factor, if you ask me, is all the things that happen beneath the hood. My team helps in sort of navigating through all of these problems and creating opportunities for the business.
So, uh, We've
Richie Cotton: got a club card program for customer loyalty. We've got online food retail and using it in finance. So these are kind of important areas. Um, I'm curious as to how this feeds into business goals. So for example, you mentioned with the loyalty program that it's all about finding out more about your customers.
How does that sort of have an impact on the business?
Venkat Raghavan: There's a strategic side to it and then there's an implementation side to it. Uh, if you look at Tesco's, um, purpose and strategy, our purpose is to, uh, help our customers, colleagues, and the planet a little better every day. It's a very humble, but a very powerful purpose.
Um, and therefore, we have taken this purpose and we have created strategic programs or themes around it. So one is around, uh, how do you make our customers love our club card program a lot more. It's called I love my Tesco club card. It's a very customer centric statement. As I said, the customer is saying that I love my Tesco club card.
Second one is on magnetic value. It's all about creating value propositions around price, promo, quality. Third is easily the most convenient, which is all your channel, sort of channels coming together to create a seamless experience. And fourth is the save to invest. As a retailer, you know, retail is a very thin margin business, especially food retail, which means that if you have to innovate on the first three strategic programs, you have to make sure that we are constantly improving and driving efficiencies in our business, uh, and putting the money back into strategic investment areas.
So these are the four strategic programs. So this is the strategic side. And therefore, data gets used in all these four programs, which eventually leads to the purpose. of helping, uh, serving our customers, colleagues, and planet a little better every day. Under each of these programs, there are strategic initiators that are analytics or data led.
Um, for example, pricing as an area. It's a big transformation program to make pricing sharper, uh, more personalized, more localized, as an example. Uh, but this is more of a five year journey. What is more operational in nature is, what does it mean on an, um, on a year by year basis? What we then do is, um, in every country, just so you know, we operate in six, seven countries, if you include India, where we have a joint venture, it's seven countries.
What we try and do is, um, make sure that we understand the commercial and the business plan of that market every year for the next 10 years. two to three years. And then we come up with a second level detail of how analytics can contribute to the sales improvement, to the margin improvement, to the cash improvement.
Uh, and this sits as an overlay in the business plan. For example, if there's a, there's a market where we want to grow our market share by say 50, 60 basis points, uh, the many teams that need to come together, but fundamentally you need analytics and science to say, how are we going to compete? Which areas are we going to grow in?
How much of investments are we going to put in there for what returns. And therefore the forecasting, the planning, the predictions, the reaching out to customers, the fighting the competition, all of that becomes an analytics work plan, which then sits very seamlessly in the first year, second year, third year.
of the plan that the business has created, which means that there are strategic intentions, but the way it lands is as an overlay into the business commitments, which I feel, you know, as the person who's leading the analytics team at Tesco, I feel tremendously proud that we have a direct connection with the business.
to what's in the business. It's not an optional, um, sort of capability. It's a very integrated capability into our business planning and business delivery.
Richie Cotton: I find it interesting that you've got these sort of multi year plans and then there's, um, like the shorter term objectives within that. So it seems like the sort of layers in terms of like going from high level strategy to like, this is what we actually have to do on a day to day basis.
Now you mentioned that, um, so there are a few different sort of areas, uh, Where this impacts the business, where data impacts the business, and improving the customer experience is one of them, sort of making people love Tesco, I guess, more through the, uh, the loyalty program. Do you want to talk me through, like, how that works?
Like, what are you doing to improve that customer experience?
Venkat Raghavan: Sure. Uh, so one of the things that I learned, uh, at Tesco, and I've been here for, uh, Now, a little over four and a half years is that, uh, uh, there's a very, uh, and everybody has a customer centricity and customer experience philosophy. I think there's no company on the planet which doesn't say that we are, or we want to be customer centric.
But what, what I sort of found really interesting about Tesco is there's a very simple, elegant definition of what that means. So what we believe is that, um, when great quality means great value. Great experiences are delivered. So that's the underlying philosophy. Quality is not just about the product.
Quality could be the quality of, um, let's say the cleanliness in the store. How quick are we able to check out from the store? It could be, uh, for example, you know, we, um, for customers who are vulnerable, um, you know, we do have the option of delivering the food to their kitchen. The people who drive our trucks can walk all the way to the kitchen and deliver the food to their kitchen.
A lot of this makes a, makes a very fundamental impact on quality of experience. Um, small things that go a long way. Um, and then this, the other side of value, which is, for what I pay, am I getting the best outcome as a customer in terms of the products and the services that I get? I think you had a question, Richie, I'll probably pause.
Richie Cotton: Yeah, so I'm curious as to how data impacts this because, um, you mentioned things like, uh, the cleanliness of, uh, of a store. Is this something that you can quantify and then go and analyze and can you talk me through how do you go about doing that?
Venkat Raghavan: Yeah, I'll probably share a little bit of the secret sauce, uh, not of all of it, um, but I think this is exactly the place where analytics and data science and AI and clear a fundamental sort of role.
Because if you look at quality, uh, quality is a very subtle, it's a perception. Um, but what you have to understand is that quality is a perception that's sort of left by the customer in different places of our business. For example, uh, when a customer buys something, um, and then they um, Return it back for whatever reason.
Uh, there's a little bit of a trail. When the customer calls us for an online order and says something, that this is great, this is not great, that's, that's a little bit of an information. Um, and when the customer takes a survey, you know, when a customer shops, they have the option of filling a survey.
That's an information that goes on what they leave on a website for a product review, it's an information and so on. So for the social media, there's a wide what they do on the mobile app, where analytics comes into the picture is, uh, is in breaking a lot of these data silos and context silos. And what we've done is, uh, you know, we try to get all of this information, whether it's contact center unstructured data.
Web reviews unstructured data, product returns unstructured data. And AI and Gen AI, we're trying to build smart intelligence to connect all of this back to a product, or a store, or a category manager, or a supplier. And all of these are insights that get surfaced to our operators. on a timely basis so that they can act on it, whether it's with the store manager or with the supplier or by changing the sort of the call patterns in our contact center.
So that's, that's where we sort of
Richie Cotton: play a role. That's really interesting. So I can certainly see how like one person yelling at you on social media is not like important stuff, but when it's part of like a larger set of like data from different sources, then that's going to be a useful sort of bit of signal.
So. You mentioned that one of your big, big challenges is having this sort of intelligence platform where you've got data from everywhere. Are you able to talk me through, like, how you've gone about building that and what do you do to make this happen?
Venkat Raghavan: So there's a, there's again a data engineering side to it, which is how do you sort of connect the data to, um, then get it into a sensible shape.
And then there's a data science and analytics angle to it, which is, you know, that things are stitched together in some sense, how do you make meaning out of it? We work very closely with the data engineering team. Uh, and it works both ways. So many times, uh, the data engineering team comes up with a third party data.
Um, they have the conversations, could be, let's say, about packaging of our products. They might be working with the suppliers to get product packaging data into our sort of, um, into our data fabric. Sometimes we learn from them saying, hey, what's, what's coming into the, uh, into the data lake. Um, uh, and then we sort of try to create a plan saying, okay, if you're getting packaging information next year, can we do something on sustainability and better?
Could we create more science around it? So that's one way of learning. The other way of learning is, uh, we identify problems. And then we work with our data engineering team saying, look, this is a problem that we have to address this year. Again, it could be pricing, promotions, quality, sustainability, whatever it is.
Then we go back and tell the data engineering team that, look, uh, Till we prove the value of this concept, we will take it sort of slightly, uh, in a more experimentation mode. We might be manually stitching data together, that's fine. But once we take it to a place where we see sustainable value, then the investments to build that into the data engineering fabric.
kicked off. So I think what is important, you know, as a learning experience, there is many times we take one or the other approach, which is business putting a lot of pressure on data engineering saying, I need this on that, or data engineering saying that, look, I'm going to go on this five year transformation program to get everything in one place.
Somewhere what's important is to have a two way communication all the time. Because things are evolving, business is moving at a different speed, competition is moving at a different speed. So this two way dialogue is, is how we build this fabric. So it's not a waterfall sequence of events saying this year we're going to do a few things and next year it's, it's, it's a constant sort of flow of intelligence both ways between the science and engineering teams.
Richie Cotton: Yeah, so this is really interesting. It just sounds like, uh, getting all your data in the right place is going to be a difficult data engineering task. So then having that communication Uh, data teams and business teams is, is going to be incredibly important. This is something I think like almost every business is struggling with.
So do you have any advice on like, how can you get that good communication flow going between data and
Venkat Raghavan: business? I think the missing link is probably the science and the analytics element. So if I were to take a step back, um, I think as industry practitioners and leaders, we know that a lot of, uh, uh, business programs sort of, uh, don't really go far because they lack the data on the Um, analytics support the same time a lot of a initiatives that we take up, whether it's genetic exploration or something that we do with traditional AI.
Many times it really doesn't cut through beyond proof of concept into the business. Uh, so therefore, you know what we spend significant amount of time in my team is to build a few frameworks, methods and mindset to bridge this gap. Um, if you have to give you an example, one of the philosophies that we very strongly believe in is, um, you know, We will avoid reaching out to business and asking for requirements.
Um, because what we feel is that many times if you go, um, and talk to somebody in the marketing and talk about how do we improve market share, given their view of what is possible, they'll most likely ask for a report or a dashboard or a few more metrics and a few more data points. Uh, at the same time, if you sit in a dark room and say, I'm going to, I'm going to crack this using genAI and you get into a 12, 24 month gestation period of R& D.
Um, Then by the time it comes out, either the world would have moved on or, uh, the problem is not even relevant. So therefore, what we always say is that business conversation should be about what is your commitment or what's your requirement. I think that's the starting point. So whether you're a data engineering team or a data science team or any other team that you call yourself, the conversation should be about what are you committing to, you know, the board, what are the numbers that you're committing to, and whether you're comfortable and whether you're not comfortable.
Because somewhere we have to understand that we're trying to enable business move forward. I think that's a little bit of a humble discussion that we need to have, saying, help us understand the commitment. And therefore, we can come back to you with the right ideas. And once you have the right ideas, it's when you need to set up the right governance and the right principles and the right controls with the engineering team.
Saying, look, we've got to work very tightly together, or the cadence of these governance meetings can be defined by. Um, the organization, but what's very important is to put the guardrails. Think, look, there's a commitment to deliver so much growth in cash this year. Therefore, what we do with finance data and what we do with finance data science.
has to have a straight line between that number that has been committed to cash improvement. So I think the fundamental principle is to go and understand commitments and try to align with commitments that are getting made as opposed to getting excited about initiatives and Uh, what's cool in the industry out there.
Richie Cotton: Yeah, that certainly just seemed important to understand like what are what are the teams that you depend on Going to commit to in order to make sure that your project is going to succeed Uh, and like there's a sort of two way dialogue there and
Venkat Raghavan: the dialogue Which is the dialogue, um has to be outcome driven Uh many times what I also feel is that if you take genAI as a classic example Uh, what genAI has beautifully done is it has You It has commoditized the concept of AI.
The good thing is that everybody is talking about AI. Uh, but what you have to be very cautious about is where does it dock? into an outcome. Um, you can always put a little bit of investments into exploration, which is outside of any outcomes, but 80 to 90 percent of the efforts of analytics or data science teams has to have a straight line between what we do and how it's moving the data forward.
Richie Cotton: Alright, so we talked a bit about, um, using data for improving the customer experience. And, uh, maybe one other important area is talking about, uh, pricing. So you mentioned that, that you have this sort of long term initiative to do better pricing. Um, what What does better pricing mean? And how do you use data for this?
This
Venkat Raghavan: is one of the most complex programs to crack when it comes to pricing because the number of dimensions that define what is good pricing, the question that you asked is fairly vast. Let me just tell you the journey. Part of this is specific to Tesco, part of this is with my general experience in retail for about 20 years.
So, 10 years back good pricing used to be, uh, You know, it used to be as simple as can you understand demand when you make change, make changes to the prices. Um, if you're able to sell so many apples today, if I reduce the price by 30 cents or 30 pence, what would that mean to the demand, the basics of pricing?
Um, And then what, what that has evolved into right now is a little bit of a digital, digitally led pricing strategy, because when you get online and if you, if you look at UK, a lot of players play online, which means that the pricing strategy can change near real time. So on one side, the speed at which we are to make price changes, especially digitally, has changed dramatically.
The second thing is pricing has also evolved from just changing the price of a particular product to see change in demand because, uh, there's a lot more happening in pricing. I think the understanding that a lot more is happening in pricing is probably a good phenomenon in the last five, six years. So just to give you an example, if, if we pick a product and if we decide to compete on this particular product, okay, everyone else who's in the market.
And if we try to reduce the product price by 20%, uh, it's always easier to model the demand for this particular product going up, uh, to what extent and all of that. But what's important to know is that a customer is not just coming to buy a particular product. If they buy a pack of, uh, chicken goujons, uh, they're also most likely going to buy French fries.
And, uh, can of coke or a Pepsi or whatever it is, which means that you have to understand pricing as a customer behavior and not as a product entity, which, uh, which means that now we are able to think of pricing as, as a basket, which means that if I promote a particular product through a price or through a promotion as a labor, what else is going to sell along with this?
Is that going to be profitable? Is that going to build the right behaviors with the customers? Is that going to drive more customer loyalty or is that going to drive more cherry picking behavior? So there's a lot more thinking of how customers are shopping as opposed to what is the price of a particular product.
So I think that's a fundamental shift in change, which means that you have to look at halo impact. You have to look at cannibalization of this product over another product. What if you sell more of a loss making product by pressing it down compared to a profit making product? So now the entire sizing size, uh, Sizing of the problem has expanded, um, to be more faster.
So this, there's a lot of digital changes that are happening. Second is also completely expanded problem. You're suddenly looking at a network of products interacting with each other. It's not a one product, one price problem anymore. It's the network. Uh, of many products in many places.
Richie Cotton: That's absolutely fascinating.
The idea that, uh, you've got to think about the price of multiple related items together as a whole. Um, and think about what, I guess, get into the, uh, customer psychology in order to understand like what they might want to buy, given the things that they've already bought or what's that, whatever's in their shopping basket at the moment.
Um, okay. So, um, in that case, uh, uh, are there any sort of specific, uh, areas or innovations in this that you're. that you currently think are
Venkat Raghavan: most important? I think pricing has been and will continue to be one of our most important levers. So, see, I think I was talking to you about, um, experience. You asked me a question on customer experience.
So there are two sides to the experience story. One is the quality side, we briefly touched upon it. The other side is the value side. Um, so we'll lastly talk about pricing as a lever in value. What we do feel, uh, what we, I mean, there are obviously a lot of regulations on how much you can sort of change your prices.
You know, how much of differentiation can you have between, let's say, your online as a channel and your store as a channel. And we have small formats, large formats. So there has to be a certain level of, again, governance and principles around which you work with pricing. But what's also important is, uh, how do you start using personalization as a, as a, as a part of the mix, price, promo, and personalization.
The price doesn't have to be just the price of the product on the shelf because then you're competing at scale. What is more exciting and what's more sort of science led now is. If you're a customer who has high lifetime value, uh, and if I know that, uh, you're not buying into our clothing, which is a, it's an extremely good category.
We are, we have great clothing offerings. You're a great customer for us. Uh, therefore, how do I use pricing as a personalized mechanism or promo as a personalized mechanism to get you to sort of try and be with us, those categories. So what's, what's happening is a little bit of, Price as a mass lever, promo as a more sort of, uh, need based lever, but personalization as a sort of underlying fabric to understand who you are as a customer and therefore how can it get you, um, into the right behaviors.
So that's, this is an area that, that we're exploring quite a bit, um, at this stage. Okay.
Richie Cotton: So, um, just to make sure I've understood this, is this the idea that you would give a customer. Yes. Okay. different promotions to different customers, uh, depending on your past relationship with them and what you know about them.
Venkat Raghavan: Yeah. So that's, uh, that's, that's the, uh, that's the summary of it. Uh, but then for us to get there, it's not just about looking at what you've purchased with us, because then you'll, you'll be getting the same offers because you've done the same thing. It's a cyclical loop. What is important is to understand, um, uh, which you as a customer, Um, you know, like I said, the future value of Richie as a customer to a business, uh, who are customers like Richie doing that Richie should be experimenting on, uh, can we understand, you know, what Richie needs that he probably does not even know Tesco has to offer because Tesco is a large company with a lot of offerings.
Uh, for example, Tesco has Tesco mobile. Uh, which many people might not know it's, it's, it's, uh, uh, consumer mobile sort of offering. Uh, we have a great brand for apparel called FNF. So many times it's, uh, it's just understanding who Richie is and therefore what do I have to offer either as a product or as a price proposition, which Richie might appreciate and get closer to me as a customer.
Um, and price and promo happens to be one of the levers to sort of personalize.
Richie Cotton: I guess the flip side to worrying about price is, uh, think about costs. So can you talk me through how data is used to
Venkat Raghavan: manage
Richie Cotton: costs?
Venkat Raghavan: The most exciting and the most, uh, talked about use cases are on customer price, promo, range, and, uh, space and retail and sometimes supply chain and logistics.
Um, But we as a retailer, um, at scale, um, you know, our single largest cost item is cost of goods sold. So which means that, uh, let's think about the math. So if we have to generate, you know, uh, a pound through, uh, through, uh, of margin through sales, we have to sell 20 to 25 pounds worth of product to generate that one pound in margin.
As opposed to figuring out a way to use science address cost, which just 100 percent translates into margin. So that's how important cost is for us. And the last two to three years, uh, uh, the macroeconomics, um, you know, have been quite volatile with tremendous amount of inflation and deflation and quite a bit of yo yo in certain commodities.
Some of our markets have gone through 40%, 50 percent inflation, which is just Unheard of in the last decade as far as I know. So what we, what we therefore try to do is, uh, is constantly look at, uh, you know, as a retailer, the disadvantage with cost is you don't exactly know what has gone into the product that you're selling.
Um, which a CPG company would know because they make the product. Um, so what we, what we therefore try to do is come up with intelligent. This is one of our biggest differentiators, and it's, it's probably something that will go for, uh, an ip, uh, for, but the construct is how do you smartly. predict the product ingredient and the mix of the ingredients, and then try to look at the commodity movement and the predicted commodity prices.
And therefore, how do you come up with a way to intelligently gauge, are you paying the right cost to your suppliers? Um, if somebody says that you have to pay 75 pence for a loaf of bread, Um, should it actually be 75 pence or should it be 68 pence? Because of the scale of Tesco, a 7 pence difference could mean quite a lot.
And if you could save 7 pence in cost, could probably reduce the price of the loaf of bread by 3 pence or 4 pence or whatever it is, and transfer that value back to the customer. This is how we think about cost and price being a very interconnected story. Um, but again, the most important factor here is you have to do this at scale.
You cannot do this for a few products in a few categories because then it's really not going to cut through into the business. So the idea is to crack the secret sauce and crack it at scale and then crack it for the group because we have, like I said, we operate in six countries. We also have a B2B business.
So therefore, delivering this for a 65 billion pound group, uh, the only way we can do this is through analytics and science. And this is one of the areas that we play very heavily.
Richie Cotton: That's, uh, pretty fascinating that you're trying to guess how much it costs your suppliers to create something so you can negotiate better on pricing.
I'm curious as to what that involves. Is it a case of reading the ingredients list, uh, like, programmatically and trying to get to that, or using mass spectrometers here and analyzing that data, like, what sort of things does
Venkat Raghavan: that involve? Richie, I have to get our lawyer involved to have a detailed discussion on this topic, because like I said, this could be, you know, a different shared IP for us, but in a nutshell, um, it's two things.
I mean, it's, one is you have to understand that, um, uh, when the commodity prices go up, uh, there's most likely going to be an increase of cost price, uh, coming through from the suppliers. So we have to be prepared to manage that conversation. And when the commodity goes down in terms of cost, we have to go proactively and ask for a decrease in cost price.
So eventually what you have to do fundamentally is, like you sort of pointed out, any product that you pick, whether it's a can of soft drink or it's a pack of Let's say bread loaf of bread, pack of chips, uh, you should be able to smartly understand from a lot of unstructured data available out there to see what are the top three, five, seven ingredients here and what's the likely mix.
Plus you also have to think about the, uh, associated costs, which includes energy, raw, you know, beyond raw materials, energy, logistics. Uh, Forex. It's a pretty large science, you know, eventually, you know, the bottom line is when you have to have impact at scale, uh, at the size of Tesco. The analytics or the data science team has to prove value through a proof of concept or proof of value.
But this thought process should be about scale, should be about different categories. It should be about different suppliers. It should be not just raw material cost. It should be the total landed cost. of that, of that product, because then you have a better chance at the negotiation table to defend an increase or push a decrease through.
So that's the high level that's as much I can reveal right now. But again, Uh, that's, uh, that's something that we do as a, as the analytics and science team.
Richie Cotton: So I think there's certainly a lesson in there for other businesses in that if you want, if you have to negotiate for like buying things from other businesses, you want to know as much about that of the business that you're buying from as possible.
Um, all right. It feels like the, um, the data processes at Tesco are fairly mature. Um, for other companies that are sort of building up their capability, I'm wondering what advice you have, like, how have you gone through this process of building up this mature data function?
Venkat Raghavan: Yeah, I think, uh, very good question.
Um, and I should thank a lot of people who, uh, preceded me, uh, in building a fantastic foundation already. By the time I joined, I think we were already at a very good place. Uh, but there are a few things that we are learning as we, as we move forward in this journey. And I, I can probably talk about what's to come.
what's relevant for other companies. One is, uh, uh, I think the success of analytics or data or AI or data science or whatever team, uh, associated with data and the value of data, um, is, is about how much impact you have created at the end of the day. So in Tesco's case, we have, uh, something we call as the big six KPIs.
It is sales, margin, cash, the hard metrics, and then colleague. satisfaction, customer satisfaction, supply satisfaction. So these are the big six KPIs for us. So one of the most important learnings for us over the last three, four years is try to be as close as possible to impacting these six KPIs. And the conversation should less be about how great Gen AI is.
It has to be more about how much Gen AI can impact one or more of these six KPIs. The responsibility and the accountability of surfacing it the right way and not in an excited way or an intimidating way has to be with the data science and the analytics team. That's probably the first learning. Uh, the second learning is once you have that level of trust sort of that level of integration into the business, how you line up your resources, you know, data science and data engineering and visualization and all of those are, if you ask me, those are all sort of, um, it's content, but it's content which is getting commoditized very fast.
Um, so the content has to help deliver whether it's a data strategy. or analytic strategy or a science strategy or visualization capability. If it is not helping you achieve those commitments in one of the big six, you're We should probably challenge it very quickly. And it's even better if the analytics team is asking itself these questions.
One of the blind spots for many analytics and data science teams is we, we refrain. It's, it's a, it's a, it's a touchy subject. Um, you know, most of the data science teams are pretty expensive teams. So the question is how much multiple are you generating in terms of, um, sales and margin and how is that measured?
Uh, and are you claiming your own victory or is someone validating your impact on them? So the second one is how do you line up your resources to make an impact? And third is I think you have to have a feedback which is both qualitative and quantitative. The quantitative feedback has to come from an independent team which is assessing, like I said, your impact and saying, hey, last year you did these 18 things, out of which six landed well, four did not, the other things I don't even know.
And therefore there is a certain level of quantitative feedback Maybe from the finance team or maybe from a partner that you work with. The qualitative feedback has to be more about, um, I think it's also subtle, you know, if, uh, if you're in a position where there's a lot of questions coming your way and you're expected to answer, that's probably maturity level one.
The maturity level two is, uh, There are also problems coming your way, in addition to questions, somebody also asking, Hey, how do I grow this? How do I reduce this? The third is, uh, somebody is coming to you and asking, Hey, I want you to help me grow this side, you know, part of the business, uh, which is a different level of trust.
And the fourth is probably you and the business partner are sitting on the same table and saying, what are we going to do together for the next three years? So this qualitative assessment of where you're on the maturities is extremely important. And if you go out of awareness on the qualitative or the quantitative evolution, I think there's always a risk of you going away from your possibilities and responsibilities.
Richie Cotton: I found it interesting that you said that the data science team ought to have some multiple of like financial value impacts on one of you, your six key, uh, Forms indicators for the financial, uh, target. So things like, uh, revenue or whatever, that feels fairly straightforward to measure. You also mentioned that you have three different satisfaction metrics.
So there was the customer satisfaction, colleague satisfaction. I think the supply satisfaction. I am curious as to like how you measure the data science teams impact against those. Like, do you have to turn that into some sort of financial value then? Or like, what, what's the benefit of, data science team proving a customer satisfaction metric by what about one point?
Venkat Raghavan: Yeah, I think so. So there are again, there are internal metrics and there are customer satisfaction survey, public satisfaction survey that happens twice a year, the supplier sort of satisfaction surveys that we do. So one is you're definitely going to hear back. There's no escaping. But what's more important is, uh, let's say we, we work on an initiative to, uh, Simplify the onboarding of a new supplier, reduce the time, um, read a lot of information, sort of help them sort of onboard very quickly, um, uh, when there's a role of data science in terms of sort of classifying them, getting them in the right place, putting them in the right part of the catalog, whatever it is, or if it's a colleague help initiator.
You want to get answers to your problems very quickly as opposed to going through multiple people where there's probably going to be some NLP and NLU and getting back with some generative answers. And same with customers. With customers there's a lot, you know, that we can do in terms of impacting their experience.
You need to have internal sort of measures to say that if I'm going to reduce the time to an answer for a colleague on payroll questions or payroll queries, there's so much. And my NLP and NLU and genAI is going to fit into that, uh, reduction of time and sort of there's a feedback score from the colleague saying, yes, I got the answer or I didn't get the answer.
Uh, the external KPIs only going to move if you're very, uh, if you're very, um, sharp on your internal KPIs. We can't wait for the ear and feedback through the survey. So we have to put internal scores to say, what are you going to reduce? Is it time? Is it ease? Is it completeness of the answer? Is it, you know, the simplicity with which you can onboard suppliers?
Is it, uh, uh, is it customers getting their deliveries on time? Is it the time that it takes to solve a problem to our call center? A lot of these will have to be internally measured. And again, these are all quantifiable metrics.
Richie Cotton: Uh, so you mentioned, uh, NLP is one of the techniques you use. Can you expand on that?
Are there any particular data techniques or data skills that you find highly valuable?
Venkat Raghavan: Yeah. So, uh, with respect to NLP, again, a lot of NLP is now neural network based. Uh, I think a modem from simple sort of bagging of words and all of that. So a lot of what we do right now is, um, a certain component of LLM.
Uh, what we are exploring right now is, uh, to see how we can take an open source LLM, but then build our own, um, sort of, uh, capabilities on top of LLM. For example, while an LLM can help understand. If the customer is happy or not, how do you classify this happiness or unhappiness as a function of product quality?
Or experience at the store or that's beyond, uh, the, um, general understanding of an LLM because then you're getting into core retail. So core, uh, let's say, you know, product as an area or sort of contact center as an area, whatever it is. So the idea is, how do we take LLM as a generic framework? It's like a generic dictionary, but then you always have a medical dictionary, and then you always have a financial dictionary, and then you always have a retail dictionary.
So what we're trying to build is those specific dictionaries, and the specific sort of NLP capabilities on top of it, which is going to help us classify, understand, solve problems much faster than, what others can.
Richie Cotton: So just being able to classify what you want to do using AI, that seems pretty
Venkat Raghavan: Sometimes you also, that what we're building on top of the LLM also has to understand, for example, if I say PGR to you Richie, I think you'll be like what is PGR?
It's up to you. It's a, you know, it's a very Tesco specific term that we use for product group reviews. It's the review that we do of our products and categories. Some of these products. Native intelligence and native capabilities will have to be built on top of a general LLM. And which is where I feel, you know, as we evolve in the next five, 10 years, where's the differentiation?
The differentiation is going to come from what you can build on top of an LLM. Uh, that's, that's the nutshell of what we have been trying to do. That
Richie Cotton: seems, uh, like a pretty fun project. Um, and also useful. Uh, so, um, so you mentioned a few times that Tesco is just like a very large scale organization. I'm wondering, uh, Dorenee.
Uh, differences like benefits or challenges that you find from doing data science at scale.
Venkat Raghavan: I mean, I, I have to start with what you just said. It's an amazing place to be and, uh, just, just think about it. We have, uh, six countries and we have 25 million customers. A lot of them are on the app. We have a B2C business, we have a B2B business, we have a mobile business, um, we have, you know, some products in banking as well.
So if, if you're a data scientist, you know, um, this is a great place to be in. And this is a company that has invested in data for the last 30 years, which means that this, this scope, this possibility, and there's also prerequisites. All in one place. Uh, so I think there are a lot of use cases in terms of connecting views between the businesses, understanding customers across our businesses, and then driving propositions into them in a very meaningful way.
Uh, but what we have to understand, uh, is that any large company, I think there's going to be two probably general, um, um, hoops that you have to jump through before you get into anything. One is the, uh, one is a fragmentation of business context because, uh, a lot of large organizations, uh, and this is a very general answer, um, are still sort of structured in a functional way.
Um, there's a CFO, there's a COO. The CEO for a country and the CMO, uh, and therefore there are populations under each of these areas. So therefore, what eventually happens at the scale of, let's say 60 billion, 70 billion, a hundred billion, 400 billion, is that the context becomes more and more sort of verticalized.
And when you try to solve a problem of how do we grow market share in a particular country, Uh, you get a very sort of, you get a very specific view depending on whom you're talking to. So it's a challenge and an opportunity is a, it's a challenge because if you are a data scientist, um, you, you cannot just it on one version of reality.
It's real, but it's one view of reality. It's one dimension of reality. The opportunity is that the data science team could become that horizontal intelligence just cutting across the organizational context and getting these richer view of what this problem really is. So this is a challenge, but also an opportunity.
The second challenge is also a fragmentation challenge, which is fragmentation of data. Because, again, it's probably something that will change in the next 10 years. But all the technology systems that were built in the last 20, 30 years, we have not built it for data science. Those technology systems are built for functional uses.
Uh, fill the form, submit the form. Um, you know, select, submit, whatever it is, right? Now, now what that has led to is a lot of, uh, Putting off data in different places, in different shapes, um, and different sizes, and also in a very functional way. The meaning behind the data is lost in many places. You might have heard of this concept of data dictionary.
Absolutely. Most of the organizations that I've worked with, a data dictionary is still not very evolved, you know, because if there's a column that says price sensitivity, the definition of that will be the sensitivity of the price. It's just not adding a lot of context to what it really is. That's because the systems are set up as functional systems.
Therefore, the data is going to be fragmented. For the foreseeable future, data scientists will have to take the responsibility of also understanding meaning behind the data. I guess most of the companies are probably just using 10 to 20 percent of the data. The other 80 percent is just dark. We don't even know what it is, and we cannot really make meaning out of it.
It could be structured and unstructured. Therefore, data scientists, You can always see it as a challenge saying, no, I don't understand this data. But the opportunity again is to say that I'm going to mix in sort of this data. I'm going to crack something through this and then I'm going to put value to this data, which has been a dormant asset.
So the biggest challenges or the opportunities are fragmentation of context and the fragmentation of data. And if you can make it an opportunity, you can probably be a winner.
Richie Cotton: Okay, fragmentation of context and fragmentation of data. So yeah, it seems like uh, certainly these are Uh big challenges once you start getting things Well, let's start working at scale.
Uh, yeah. Those are those are things to worry about for anyone in a growing organization Um, all right. So before we wrap up, what are you most excited about in the world of data?
Venkat Raghavan: Okay, there are many things to be excited about. I just feel I have so much gratitude To begin with Richie that I I you know, I got into this
Java developer. I just so happen to get into this industry and I've been in this industry. There's so much to be happy about. One is, uh, you know, I always say that, um, The space has four C's, and if you have to be successful, people ask me, you know, how do you spot exceptional talent versus good talent? I always tell them it's a combination of four C's.
It is content, context, commerce, and culture. And this is not dependent on whether you're a new joiner or you're 20 years experienced. It doesn't really matter. If you are fascinated about your skills, I'm an expert in we and suddenly we have like a million genAI experts the technologies of a year old, but we still have so many experts in that space.
You're fascinated about a particular skill, you're a content person, it's good for you. But you've got to understand that, you know, by. My niece, you know, she's in eighth grade, she learns Python and she has AI as a paper in eighth grade in India. So, increasingly, content is going to get commoditized.
Context is about, what is the real problem? Who are the real people? What's the real agenda? Am I dependent on somebody to give me a problem or can I find something and can I do something meaningful? That's all context, the context of where you are. The third one is commercial value. You know, the, uh, the real.
Impact is a real impact and it has to be tangible as much as possible. The fourth is culture. Can you build the culture of data driven thinking? Now, if you have to think about what I'm excited about, I have always maintained this framework. I'm very excited to see this play out in front of my eyes.
Content is becoming commoditized. Gen AI is just, you know, in the next three years, I think data science will be written through Gen AI. You just have to tell what the, uh, the algorithm is, what you're trying to minimize, maximize, what are the constraints. And I think the technique selection and writing off the best possible code with the narrative on the, all of that will be done through Gen AI.
I don't doubt it. Which means that context, commerce, and culture is where we're going to shift to. And I'm very excited to see how we're going to do that. First of all, we cannot let. AI or Gen AI laws, you have to put a lot of controls and frameworks and governance. But then if you can sort that out, then I think we can build a lot more business relationships.
We can find new propositions, a new way of growing ecosystems. Everything is becoming an ecosystem business, right? So how do we make ecosystem businesses in a more networked way? And how do you make sure that eventually we all learn how to coexist and either to get threatened or to go overboard in terms of culture of decision making?
So I feel. I feel extremely excited about all of this playing out in front of my eyes and I have to be just a part of the journey.
Richie Cotton: Uh, yeah, that's a great say to be just excited about a lot of things. I feel exactly the same way. Uh, so yeah, brilliant. Uh, thank you for your time. Thank you. Rishi,
Venkat Raghavan: a pleasure talking to you and, um, thanks for building the industry.
podcast
How Walmart Leverages Data & AI with Swati Kirti, Sr Director of Data Science at Walmart
podcast
Monetizing Data & AI with Vin Vashishta, Founder & AI Advisor at V Squared, & Tiffany Perkins-Munn, MD & Head of Data & Analytics at JPMC
podcast
Scaling Data Engineering in Retail with Mo Sabah, SVP of Engineering & Data at Thrive Market
podcast
Data & AI Trends in 2024, with Tom Tunguz, General Partner at Theory Ventures
podcast
[AI and the Modern Data Stack] Adding AI to the Data Warehouse with Sridhar Ramaswamy, CEO at Snowflake
podcast