[Radar Recap] Scaling Data Quality in the Age of Generative AI
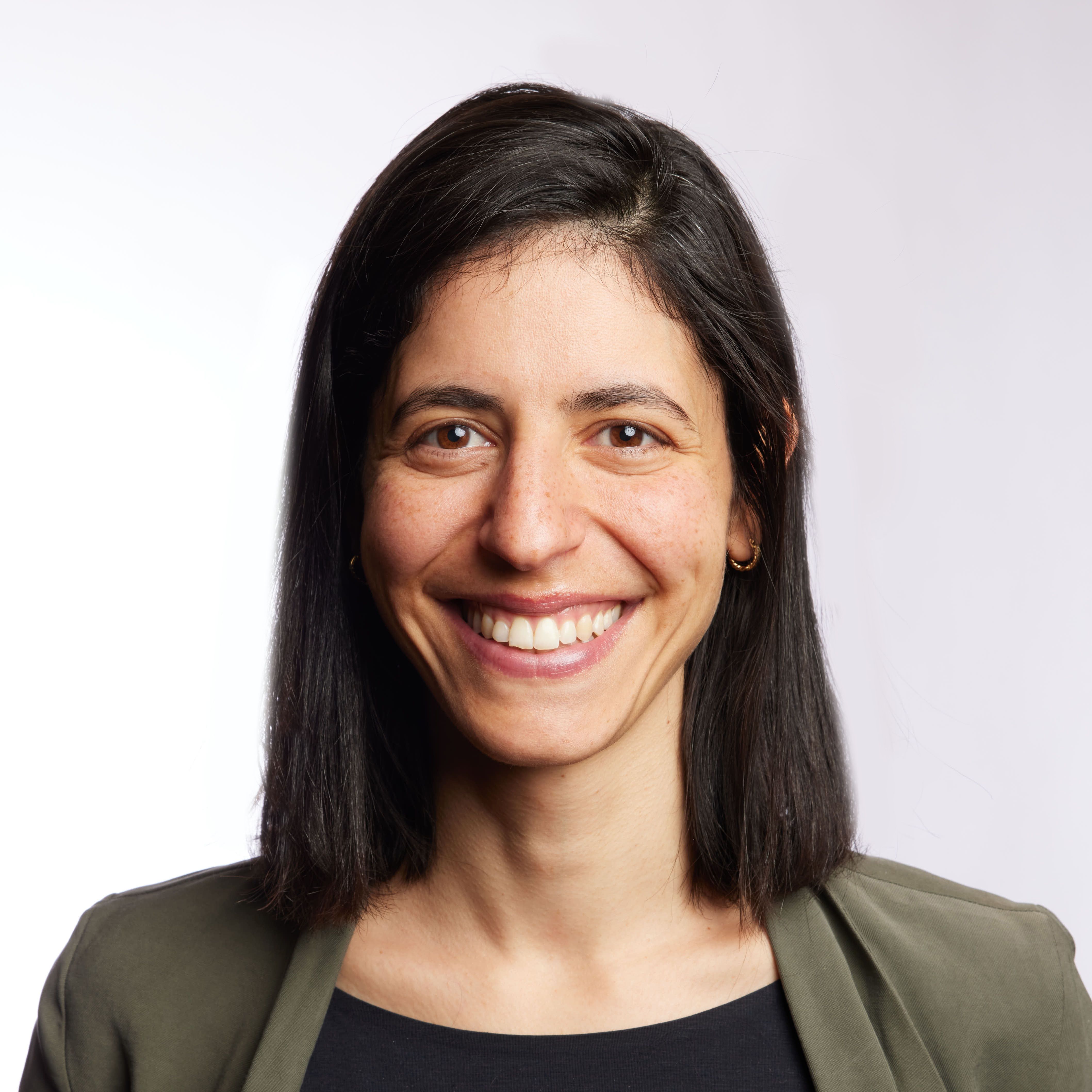
Barr Moses is CEO & Co-Founder of Monte Carlo, a data reliability company backed by Accel, GGV, Redpoint, and other top Silicon Valley investors. Previously, she was VP Customer Operations at Gainsight, a management consultant at Bain & Company, and served in the Israeli Air Force as a commander of an intelligence data analyst unit. Barr graduated from Stanford with a B.Sc. in Mathematical and Computational Science.
Prukalpa Sankar is the Co-founder of Atlan. Atlan is a modern data collaboration workspace (like Github for engineering or Figma for design). By acting as a virtual hub for data assets ranging from tables and dashboards to models & code, Atlan enables teams to create a single source of truth for all their data assets, and collaborate across the modern data stack through deep integrations with tools like Slack, BI tools, data science tools and more. A pioneer in the space, Atlan was recognized by Gartner as a Cool Vendor in DataOps, as one of the top 3 companies globally. Prukalpa previously co-founded SocialCops, world leading data for good company (New York Times Global Visionary, World Economic Forum Tech Pioneer). SocialCops is behind landmark data projects including India’s National Data Platform and SDGs global monitoring in collaboration with the United Nations. She was awarded Economic Times Emerging Entrepreneur for the Year, Forbes 30u30, Fortune 40u40, Top 10 CNBC Young Business Women 2016. TED Speaker.
George founded Fivetran to help data engineers simplify the process of working with disparate data sources. He has grown Fivetran to be the de facto standard platform for data movement. In 2023, he was named a Datanami Person to Watch. George has a PhD in neurobiology.
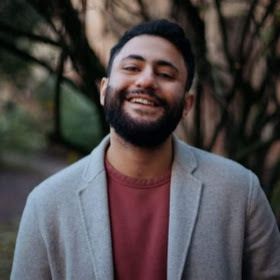
Adel is a Data Science educator, speaker, and Evangelist at DataCamp where he has released various courses and live training on data analysis, machine learning, and data engineering. He is passionate about spreading data skills and data literacy throughout organizations and the intersection of technology and society. He has an MSc in Data Science and Business Analytics. In his free time, you can find him hanging out with his cat Louis.
Key Quotes
While we've become a lot more sophisticated with what we demand from our data and from our data infrastructure, we have not become more sophisticated in how we manage data quality.
You can never actually get to 100% quality, you just have to manage it. You have to identify the highest priority areas where it is most important that the numbers be right and prioritize those.
Key Takeaways
Foster collaboration between data producers and consumers. Shared awareness and understanding of data processes can bridge gaps and enhance data quality.
Implement automated solutions for data quality checks to replace manual processes. This can significantly reduce errors and improve the efficiency of data management teams.
In the era of generative AI, proprietary data is a key differentiator. Ensure your proprietary data is high-quality and well-managed to maximize its value in AI applications.
Transcript
Adel Nehme
All right. All right. All right. Hello. Hello everyone and welcome to the final session of the day of data Camp radar on scaling data quality and the age of generative AI we left the best for last so everyone do give us a lot of love in the Emojis as you can see here.
00:00:17
Adel Nehme
Below and let us know where you're joining from. I see more than 500 people in the session already. So yeah, do let us know, uh where you're joining from, uh, especially and what you thought data Camp radar the way 1 and why you're excited about data Camp radar Des 2. Um, so of course organizations continue to embrace Ai and machine learning the importance of maintaining high quality data has never been more critical and there are arguably no better people in data Data Business across the board than bar Moses for comp and George Frasier to come talk to us about data quality. So first, I'm going to introduce bar Moses. She is the CEO and co-founder of Monte Carlo a pioneering company in data reliability and the creator of the data observability category Monte Carlo is backed by by top VCS such as XXL ggv redpoint iconic Road Salesforce Venture and ibp part to see you.
00:01:09
Barr Moses
Thanks for having me.
00:01:11
Adel Nehme
Next up is prakalpa Sankar. She is the founder of atlan proa is a leading modern data and AI governance company on a mission to enable better collaboration around data between... See more
00:01:35
Prukalpa Sankar
Thanks for having me. I'm excited for this.
00:01:37
Adel Nehme
awesome
00:01:39
Adel Nehme
And last but not least is George Frazier CEO at 5 Tran, uh, George founded 5 friend to help data Engineers simplify the process of working with disparate data sources. He has grown 5 front to be the de facto standard platform for data movement and 2023. He was named the data Nami person to watch. He also has a PHD in neurobiology George Gio
00:02:01
George Fraser
Great to be with you.
00:02:02
Adel Nehme
And just a few housekeeping notes before we get started. There will be time for Q&A at the end. So make sure to ask questions by using the Q&A feature and vote for your favorite questions. If you want to chat with the other participants use the chat feature, we highly encourage you to engage in the conversation. If you want to network and add folks on LinkedIn and share your LinkedIn profile. They will be removed automatically, but do join our LinkedIn profile that is linked in the chat as well and you can connect with fellow attendees and I think this is a great starting point to start today's session. Um, you know stay safe to say that data quality is at the top of Mind of many data leaders today, especially with the generative AI boom that we see, um, but maybe to set the stage how would you describe the current state of data quality within the industry within organizations today? And what do you think are the common challenges organizations are facing with when it comes to maintaining high quality data bar. I'll actually start with you.
00:02:54
Barr Moses
Sure. I have lots of opinions on this topic trying at the hog the entire time. Um, yes data quality. Well frankly. Let me start by saying it has been a problem and an issue in the space for the last couple of decades. So nothing is New Right. We've been complaining about data quality for a long time. We shall continue to get to to complain about the quality of our data for a long time. Um, however, I do think a few things have changed, um, first and foremost, you know, obviously the generative AI products, you know, being more, um, uh are being prevalent, uh, at least in terms of the desire to to build them. Um, uh data teams are put under a lot of pressure. Um, we actually put out a survey that showed that we surveyed sort of a bunch of data leader data leaders and 100% of data leaders were cited as um, uh Under Pressure to deliver generative AI products.
00:03:47
Barr Moses
Uh, no 1 said they are not being asked to build something. Um,
00:03:50
Barr Moses
However, uh only 70% of them just under 70 68% of them actually feel like their data is ready for generative AI.
00:03:58
Barr Moses
So that means that while there's a ton of pressure from sea level and board and others in the market to actually build the generative Ai No 1 or the large majority of people don't think that their data is ready ready for that. And I think that poses a good question for us as an industry to figure out why that is the case. Um, and my hypothesis is that
00:04:19
Barr Moses
what I would call the data state has changed a lot in the last 5 to ten years. So the way in which we process transforms store data has changed a ton but the way in which we manage data hasn't changed at all. And so that means that you know, if you go back to the survey actually 50% of um, those data leaders still use manual, um, sort of approaches to data quality. And so while we've become a lot more sophisticated with what we demand from our data and from our data infrastructure, we have not become more sophisticated in how we manage data quality. Um, you know, I think manual rules will and always be important but that there are not the end all be all. In fact, it is just the starting point. Um, and so I think the you know in short if I had to respond to what is a state of data quality today, I think there are new Pro. It's sort of an old problem with new challenges that we have not cut up yet. Um, definitely have ideas on how
00:05:17
Barr Moses
How we need to solve that uh, but I'll pause that uh, I'll pause there for a minute and see if any reactions from my esteemed, uh fellow panelists.
00:05:25
Adel Nehme
I'll let you react here.
00:05:27
Prukalpa Sankar
Yeah, I think I mean I agree with everything that far said but I I think that 1 thing to like abstract this a little bit over like I think about this concept of Data Trust.
00:05:39
Prukalpa Sankar
More than just data quality or like and maybe this is the reason you have the 3 of us in this panel. But like you know the way I think about this is like
00:05:46
Prukalpa Sankar
if you think about that final layer of trust, uh, and you have a human who says this number on this dashboard is broken. Oh my God it or it doesn't look right. Like what's wrong, right? It sounds like a very simple question. It's actually a very difficult question to answer because the reason a number could be off could be because the fight fighter pipeline that day broke and then run it could be because uh somebody device
00:06:06
Barr Moses
That never happens. Pulpa. What are you talking about?
00:06:08
George Fraser
What are you talking about take off this panel?
00:06:12
Prukalpa Sankar
or
00:06:14
Prukalpa Sankar
fail, right
00:06:15
Barr Moses
Never happened.
00:06:18
Prukalpa Sankar
Or it could be because it could be because the data quality checks that day failed it could be because someone changed the way we measure an annual recurring revenue and like No 1 forgue like No 1 remember to update the data consumer, right? And so if you think about this flow, I almost think of it as you have data producers who actually kind of want a guarantee trustworthy Self Service day like no data producer wants to spend their time answering the question of why your number is off and on the other hand like you have data consumers who actually want
00:06:48
Prukalpa Sankar
Do use data like No, 1 actually cares about the quality of the data. Like they actually just want to use the like a data consumer cares about making business decisions.
00:06:54
Prukalpa Sankar
And in the middle we have this Gap and the reason we have this Gap is because we have a prolific it it's almost self tra problems. We have created, uh significant number of tools that have been that that have scaled massively but we have a proliferation of tools. We also have significant diversity in people. So any single final dashboard probably at 5 people touch touch it
00:07:16
Prukalpa Sankar
This problem just gets worse in the AI. Yeah. So at least if I was a human I look at the number and I'm like, oh, maybe the number doesn't look right and I can do something about it. If I'm AI I don't do that and that can actually like lead to pretty significant.
00:07:29
Prukalpa Sankar
So I think the way we think about this.
00:07:31
Prukalpa Sankar
Uh, and we sit on that layer between the producers and the consumers and bringing this stuff together is what does it mean to create these data products finally, like what makes something reusable and trustworthy. Uh, and how can you bring context across from the pipeline from data quality from all of these layers in the stack like human context to solve the trust problem or the gap?
00:07:55
Adel Nehme
Okay, that's really great and George. I'll let you react to you.
00:07:59
George Fraser
yeah framework is right, um that
00:08:05
George Fraser
There's a lot of layers to the system and it matters a lot where the problem is arising except the part about 5G breaking that Network.
00:08:12
George Fraser
No, but I mean you would be you would we we try very hard to avoid contributing to the data, uh quality problem in our layer. You would not believe the amount of effort that goes on behind the scenes to try to chase down the long tail of replication out of sync buds that can happen with all the systems we support.
00:08:32
George Fraser
Um, we are not perfect. I can only say that we are better than everyone else. Uh,
00:08:37
George Fraser
so I I think where it happens is very important in terms of
00:08:41
George Fraser
Troubleshooting. Um, you asked like why despite all the efforts in this are is this? Um,
00:08:48
George Fraser
Who uh, you know created an account on your website so you can never actually get to 100%
00:09:28
George Fraser
Quality, you just have to manage it. Uh, and you have to identify. What are the what are the highest priority areas? Where is it? Most important that the numbers be right prioritize those work on those. Um, but you you I think you've got to start out acknowledging it will never be perfect.
00:09:43
Prukalpa Sankar
Yeah. Yeah. No, I I agree. I I think the thing on that is kind of what you said right that I think things will always like the reality of running like especially real time Dynamic data ecosystems is that things will always break like there's it's likely that there will always be things that are because it's that like, it's just the nature of the Beast.
00:10:01
Adel Nehme
Mhm.
00:10:02
Prukalpa Sankar
And so that's why I think a lot of our next when you're thinking about trust trust doesn't actually break because something went wrong.
00:10:07
Prukalpa Sankar
Trust breaks because someone told you your stakeholder told you that something went wrong without you telling them actually something went wrong today. Maybe you should like and I think that's the element of trust which is its 1 of something and
00:10:23
Prukalpa Sankar
I don't think the solution is trying to make sure nothing ever goes wrong. The solution is how do you go 1 level above and make sure that you solve for trust and then how do you measure and manage it over time?
00:10:32
Adel Nehme
I think that's sorry continue.
00:10:32
George Fraser
Yeah, trust trust is a good word and it is it is very hard to win and very easy to lose an example of this. I heard a long time ago. It's funny. I'm in New York right now and I met with somebody earlier today at Bloomberg. Actually. I still have the iced coffee that I got a lot.
00:10:49
George Fraser
Was there uh and long time ago. I don't know if you know Bloomberg is but they do data feeds. Um,
00:10:56
George Fraser
Finance um
00:10:56
Adel Nehme
Mhm.
00:10:57
George Fraser
What kind of data management um, but it's it's data that uh, you know, like stock prices commodity prices gas prices things like that many years ago when 5 Trend first started 1 of the things I learned is a key element of their business is that uh, the um is that is not is is that it is accurate even in these obscure cases, you know, the price of beans and Korea or whatever it is, like even the most obscure data feeds they are more accurate than anybody else and that is really important because if 1 thing is wrong 1 day out of the year that is a huge problem. That's something we've always tried to emulate at 5 Trend in a very different context replicating a company's own data, but it it speaks to how it is. When you when you're in the when you're in any kind of data business. Um, you can you can be the difference between zero errors and 1 error is bigger than the difference between 1 error and an Infiniti, uh trust is so hard hard to win and so quickly lost.
00:11:53
Adel Nehme
And and by late react and then I'll ask my next question.
00:11:57
Barr Moses
Oh, I was just going to say just reflecting on this like I wouldn't be surprised if we would be seeing in a panel like in 10 years from now still having you know, sort of similar discussions except the words change and definition change. So maybe we call it, you know trust or data quality or whatever now like hallucinations and the context of generative AI right. Um, but the problem Remains the Same, um, I think 1 of the the sort of interesting questions to to answer is like what are to think there's like what what are our, you know customers and who are now faced with
00:12:28
Barr Moses
What are the challenges that our customers today are faced with and how are they dealing with that? And how is that different from you know a few years ago or honestly just like a year ago. Um, and the reality is like these problems are just not going away and so figuring out how to address those. Um, you know in a way that uh adapts to where our customers are and meeting them where they are is is uh, I think super important
00:12:51
Adel Nehme
Earlier in discussion is that same problems different challenges? What are those challenges today? So I'd love to learn that from you.
00:13:26
Barr Moses
Yeah, great question. And I mean I'll start by saying look like if you know.
00:13:31
Barr Moses
You could in some world, like if the model output is wrong or you know, you sort of you know, you you're prompting with a question and and in the answer is is wrong. Is it better to to not have an answer at all? Like is no data better than bad data. Um, maybe I I think so, but also then what's the point of having, you know kind of a Q&A or a chatbot if we can't provide you an answer at all, right and so like
00:13:58
Barr Moses
To your question the definition of good. What does good look like actually becomes tricky. Um, and how do you define? Like, what should we strive for changes? I think um, but you know to your particular question, like what are the challenges or kind of pinpointing those? Um, I think you know kind of kind of how I'm alluded to sort of how the data state has changed over time. I think the historically what we've done, you know, when it comes to sort of trust to prakalpa point was really start with can we figure out about data issues before anyone else Downstream learns about it? Right? Whether that's you know, in in in generative AI or not, whether it could be in a dashboard. Um, and so, you know, the the the thought is that if you know, we can catch issues before others Downstream do we can sort of either repair that trust or rebuild that trust? Um, I think what we're seeing right now is that the challenge that is definitely a very important challenge to um to address and I think the detection capability
00:14:47
Adel Nehme
Mhm.
00:14:58
Barr Moses
is have evolved a certain degree, you know, I sort of talked about manual solutions for that versus not I think sort of the big kind of like next leap here for building, you know, data quality Data Trust, whatever you want to call it. Um is sort of going Beyond detection and taking the next step of sort of understanding. How do you actually resolve how do you actually address these problems? Um, and when you think about the root cause of these challenges that has changed too and so in the past like, you know, you really
00:15:20
Adel Nehme
Mhm.
00:15:28
Barr Moses
And I think if you think about sort of the core pillars of what makes up the kind of like data State I would call it. There's 3 things the first is the data itself. So actually like the data sources whatever, you know, kind of you're ingesting. The second is uh the code so, you know code written by Engineers machine learning Engineers, uh, data scientists, uh analytics Engineers Etc. And then the third component is the systems or the infrastructure basically the jobs running all of that.
00:16:05
Adel Nehme
Mhm.
00:16:19
Barr Moses
And so you have multiple teams multiple building multiple complex webs of all 3 of those things. The problem is that data can break as a result of each 1 of those 3, so it could be as a result of the data, you know, just that you ingested being totally incorrect. It could be the result of you know, bad code bad code could be like a bad join or a schema change or it could be a system failure. I won't name names but systems do feel do fail, right? It could be any elt general elt solution that you use and so actually like understanding that um, in order to really build reliable products, you have to look at and understand each each of those components you first of all have to have an overview and sort of visibility in each of these components and then also understand can you correlate between a particular data issue that you're experiencing and say a code change or an infrastructure change or anything like that that is really really hard to do today. Um, and so what ends up happening is that data teams are in dated with lots of you know alerts.
00:16:40
Adel Nehme
Mhm.
00:16:54
Adel Nehme
Mhm.
00:17:20
Adel Nehme
Mhm.
00:17:21
Barr Moses
Store kind of you know, get a quality detection data quality issues and they're all flying around between you know, 20 to 30 different data teams and 10 different domains and go figure like who needs to address which problem so in or which alert, um, so you're actually like, you know down to like the brass tacks of how do we handle this? Those are some of the challenges that I think really sort of figuring out
00:17:41
Barr Moses
How do we both have really strong detection of issues but then how do we go to the next step and actually figure out what is a root cause and honestly often times it's more than just 1 root cause so it's typically, you know, this shitstorm, excuse my language with like every single thing breaking right? Like it'll be both a data a code and a system issue. Um, and so, you know, when I think about how our systems can get more sophisticated or how we build more reliable, um, Data Systems, it has to have a more sophisticated view of what's actually um, uh, what are the, you know various components of that and what could break
00:18:16
Adel Nehme
That's really great and maybe George Premier perspective adding on top of what Barr said. What are the challenges that you're seeing today? When it comes to scaling data quality or like, you know, improving moving the needle on data quality.
00:18:31
George Fraser
Well, I mean we look at a very particular slice of this we look at the replication piece. Does the data in the central data warehouse match the data in the systems of record be it. Um,
00:18:43
George Fraser
A a database like postgres or Oracle or a app like Salesforce or workday? Um, and we you know, we we've we've come a long way with uh,
00:18:57
George Fraser
And and and squash data Integrity issues. We are experimenting with some new ideas to try to get that last little bit that last 0.1% is very hard. Um, and they include uh, the the most uh, exciting idea right now is the idea of doing direct sampling for validation. Um, so you know from when you're when you're in the business of replication data quality can be seen as you basically just need another sink mechanism that you can use to compare against uh, and um, we have we've done a few iterations internally, um, we've we've shipped things and these these are all running in the background. These are not things you see as a 5 track customer. Um, and we're we're basically we pull samples of data from The Source or the destination and compare them, uh to just create a totally out of band mechanism to verify and we've discovered for example, we discovered a floating Point truncation bug when we write CSV files for loading in the data warehouses by doing this. Um, and we think there are more things out there, uh that we could we could discuss
00:19:02
Adel Nehme
Mhm.
00:19:19
Adel Nehme
Mhm.
00:19:38
Adel Nehme
Yeah.
00:20:01
Prukalpa Sankar
it's
00:20:11
George Fraser
Discover and fix by doing that and then the other side of this is at some point we want to make these capabilities customer facing because there's a lot of phantom data Integrity issues in our world. We get a lot of reports from customers where they're like, oh my train is broken. This system doesn't match and sometimes they are right. Uh, we do occasionally have bugs but a lot of the time
00:20:17
Adel Nehme
Mhm.
00:20:33
George Fraser
They're they're compared their there's something wrong with the the comparison that they're doing. And that that doesn't mean that we just tell them to go away. We have to figure it out. We have to verify that it's a like a false alarm. So we get a lot of false alarms of 5 trans. So the event that we can build tools for quickly, um, proving or disproving the the concern where we're thinking about that.
00:20:44
Adel Nehme
Yeah.
00:20:52
Adel Nehme
That's awesome and and Pablo from your side of the you know, data quality Island. What are the challenges that you're seeing today?
00:20:58
Prukalpa Sankar
Yeah, so the way I think about it is I think of it as a 3 step framework. Uh, it's actually very similar results. I think like generally like Life Health. Um, it's awareness. Um, that's the first step the second step is cure.
00:21:07
Adel Nehme
Mhm.
00:21:13
Prukalpa Sankar
Uh, and the third step is prevention.
00:21:15
Prukalpa Sankar
uh
00:21:17
Prukalpa Sankar
To use us with 5 transaction and I think like for example 5 10. We were like the metadata API that came out now we have customers that say let's pull out.
00:21:34
Prukalpa Sankar
Context on what's Happening, um and send out an announcement directly to my end users, which is red green yellow. Is that with the pipeline run or did it not run did it run as I expected stuff like that? Uh, we have the same thing with anomaly detection on the so the stuff that the data producer is now can we share awareness to end consumers and end users and in a way that's easy for them. It's in their bi tool. It's in slack. It's the slight green announcement that says red green yellow, right like stuff like that. That's first step. Can we create awareness of where we are the 1 big change we've seen because is this move to this concept of a data product where I think some of the most furthest ahead teams are actually taking all these metrics and metadata and converting it to almost into a score which is a data product score and it says like here like, let's create a measure of like, you know, if you don't measure you can't really improve what's the measure of the usability and Trust as I think about a data product. So that's been I I mean, I've been super surprised by how quickly that adoption has grown.
00:22:05
Adel Nehme
Mhm.
00:22:35
Prukalpa Sankar
across our customers
00:22:38
Prukalpa Sankar
The second on Cure Car alluded to this collaboration. I think that's the most broken flow that exists right now because cure is a solution between business and data producers both need to come together.
00:22:48
Prukalpa Sankar
So there's a mass like there. I think we have a lot of work to do when we come back maybe not in 10 years like we come back. I can even like a year like we've made significant progress in collaboration.
00:22:56
Adel Nehme
Mhm.
00:22:59
Prukalpa Sankar
And the third is prevention. I think the biggest piece here like we're seeing a lot of adoption around data contracts and preventing.
00:23:06
Adel Nehme
Mhm.
00:23:07
Prukalpa Sankar
So, how do you take?
00:23:09
Prukalpa Sankar
What you learned in awareness and cure it but also make it something that's more sustainable over time. And I think that that's actually where there's been a a bunch of innovation. I think like we launched a module but there's been a ton of innovation over the last uh the last some time um, and hopefully all those 3 things together actually get us to a point where we solve for data trust in. You know, I really my vision for this is like in a few years like it becomes a really boring problem. Like we're not talking about it. It's like it just it's there. Uh, and then we keep improving it but it's not a it's not a problem that we should have a topic of conversation about it should become stable sticks.
00:23:47
Adel Nehme
yeah, and
00:23:48
George Fraser
What do you mean by data contracts?
00:23:52
Prukalpa Sankar
Of this is how do you help a producer and a consumer a line on an SLA? That's the best way that we're looking at and so
00:24:02
Prukalpa Sankar
What do you believe are the core rules for data quality again? It's it's a little bit more of a collaboration problem actually more than it's a technical problem, which is what is what do we agree on is our core layers of this is what we believe and then how do you translate that into the actual data producer workflow itself? That's the best example of what we're seeing um customers turn the center.
00:24:28
Adel Nehme
And there's 1 thing that you mentioned for KA which is on the collaboration side that I think is very very important, which is that data quality often a cultural issue as much as it is, you know broken pipelines or like, uh, you know, something happening on the data collection side. Um, can you walk us through maybe the main call issues that lead to poor data quality like an expand like that notion a bit more like what can happen on organization what can organizations do today to shift their culture to prioritize their quality so that you lead with the Ault and then I'd love to listen in front of that remaining finalists.
00:25:02
Prukalpa Sankar
Yeah.
00:25:03
Prukalpa Sankar
Every cultural thing right? I actually think of it similar like what's the base of culture like first if you believe in like if you think about if you believe in good intent, which I would like to believe that most like everyone actually is trying to do the right thing for the company to a large extent right like
00:25:18
Prukalpa Sankar
No, no, like no data producer wants to like ship something that's like breaks and then spend time like nobody wants to work. Like let's let's start like everyone was like everyone wants good in that.
00:25:27
Prukalpa Sankar
Um, so I think the first step is really I think just shared awareness and shared context.
00:25:33
Prukalpa Sankar
Uh, so first like a lack of like I remember this I remember once I got like I used to be a data leader in my previous life and I got this call from a stakeholder and they were like number of this dashboard doesn't look right. I remember like jumping on my bed and I look at my dashboard and there's a 2X Spike overnight and I'm like, oh my God, this is crazy. And even I in that moment had a question on like, oh my God, it's something break or is my data engineer not doing his job.
00:25:56
Prukalpa Sankar
And even I had that because I couldn't just open up airflow and look at the audit logs and see what happened. Like I just couldn't so first step the reality. Is that 5 different people with their own DNA?
00:26:11
Adel Nehme
Mhm. Mhm.
00:26:15
Adel Nehme
Mhm.
00:26:25
Prukalpa Sankar
Uh, which is why I'm super excited because if you create a measure then it becomes very easy for people to move to it. So I think as you think about so how do you measure it is the second um, and then the third is actually then just the process flows its tooling process flows, like iterative Improvement. That's actually the easy part of the problem in my mind. I think the first 2 things like for example even shared context J said this right like you break.
00:26:31
Adel Nehme
Yep. Yep.
00:26:44
Adel Nehme
Mhm.
00:26:49
Prukalpa Sankar
It's very easy to lose trust.
00:26:52
Prukalpa Sankar
Uh, but at that time, nobody says 99.5% of the time it was accurate. You see the 1 time the number was broken and it breaks trust right? And so then what's the shared understanding? What are we defining as Trust?
00:27:05
Prukalpa Sankar
And how do you solve that human problem? Um, I think the best examples of this is we've seen people actually have folks who understand both culture and humans and Data Drive the charge on building that initial people calling covenants standards people whatever you decide to call it, but that initial share context and understanding as I think the first step to good culture.
00:27:28
Adel Nehme
Yeah, and I'll let George I'll let you react to that like maybe what are some of the levers that you've seen that can improve on cultural level to improve data quality within an organization. Maybe kind of getting inspired from 5 train customers here.
00:27:40
George Fraser
oh my gosh, I'll let you know when I find 1, uh
00:27:46
George Fraser
Unfortunately, a lot of data quality problems Have and Have origins in like poor systems configuration, and those things are really hard to fix. Uh
00:27:57
Adel Nehme
Yeah.
00:27:58
George Fraser
You know, um, if I have 1 piece of advice for early stage Founders it is keep an eye on your sales force configuration because if that thing gets out of joint, uh, man, it is hard to fix. Uh, so it's it's it is a real grind trying to make progress on this a lot of it consists of going Upstream to the systems of record and improving their configuration so that they're not generating like a zillion duplicate accounts and stuff like that.
00:28:28
Adel Nehme
Yeah, I can attest to that I can attest to that and then uh bar from your perspective culturally, maybe how do you move the needle as a data leader?
00:28:33
Barr Moses
Yeah.
00:28:36
Barr Moses
I mean agree with what peraba and George said maybe the respective that I can add here. I think the companies that we are seeing make progress. It's due to a few reasons. The first is there's both this organizational top down and bottom up.
00:28:52
Barr Moses
um agreement that data matters
00:28:55
Barr Moses
And that the quality and Trust of that data matter, um, if it's just 1 direction that typically fails. Um, so if there's you know,
00:28:60
Adel Nehme
Mhm.
00:29:05
Barr Moses
you know, there's like a CEO of 1 of the you know, Fortune 500 Banks gets upset every time uh, they get a report with bad data and so they actually made it a sea level initiative to um,
00:29:19
Barr Moses
To make sure their data is is sort of you know, Ready Clean to the best degree that they can Etc. Um that obviously creates some pressure creates, you know, real initiatives in the business real metrics to process Point earlier. Um, that is not sufficient. Um, it's very important but the, you know, sort of business teams if you will business analysts, but also the data governance teams, um in large Enterprises, there's various, you know, it could be the centralized data engineering platform all those people need all the different teams and um are all stakeholders in an
00:29:24
Adel Nehme
Mhm.
00:29:53
Barr Moses
Initiative and need to care about it just as much for those teams oftentimes the motivation is that their spending most of their days in fire drills on data issues. Um, and by the way, I saw someone sort of asking can we clarify? What is a data issue? I think that's a great question. Um bad bad data can look in various forms and has you know can can you know, its symptoms are are so different. Um, but generally what you know, the way that that I'm thinking about this is if you look at some data product, whatever data products that is again, it could be um pricing recommendation. It could be you know,
00:30:08
Adel Nehme
Mhm.
00:30:27
Barr Moses
A dashboard that your CMO is looking at um, it could be a chatbot. And if you're looking at it you look at the data and it's very clear to you that the answer is wrong. Um, maybe the most, you know, an example for the last few weeks. Um from this was from Google I think someone searched. How do you how do I keep my cheese on my pizza or something like that and Google recommended you can use organic superglue. That's a great way to keep your keys and if you
00:30:51
Adel Nehme
Oh, that's like a chance of search from Reddit. Yeah.
00:30:54
Barr Moses
Yeah, exactly. That's yeah exactly. That's right. And so, um that is a good example of bad data, um that you know, that is 1, you know a very public and I think it went viral and you know, maybe Google Google can get away with that but many other companies can't get away with that. So there was um, you know an airline that actually um provided the wrong uh discount on an airline ticket and so, you know consumer purchased a ticket at a different price and actually sued that Airline and got the
00:31:24
Barr Moses
Got the money back. We keep the money. Um, and so, you know, they're really real repercussions to putting bad data out there. Um, and I think you know going back to your question about culture. I think you know both
00:31:37
Barr Moses
The the teams working with data have to care about that. Now the thing is that they don't always do because they don't always understand. Where is your data going? So if I'm building a data pipeline, I don't necessarily understand who's being who's using that data. Um and why which makes sense if I'm way upstream and so often times I find that um, the companies who have made the most progress are those are able to bring together those teams under a unified view of where we want to go as a company, um oftentimes that could start looking at just how many data incidents do we have? Um, how quick are we to respond? Like what's our time to to detection of those? What's our time to resolution and then, you know taking this a step further, uh, oftentimes our teams putting together slas between each other so, you know the SLA for particular data to arrive on time or to arrive in some complete State. Um Etc.
00:32:02
Adel Nehme
Mhm.
00:32:28
Barr Moses
So I would say kind of the focus on metrics. I agree with prakalpa that that's um that typically drives the right Behavior or drives some Behavior, which is better than none.
00:32:37
Adel Nehme
Okay, I couldn't agree more and then maybe you mentioned something bar that I'm gonna.
00:32:40
George Fraser
On metrics will drive some Behavior. I agree with that. I agree.
00:32:45
Adel Nehme
I'm gonna get that tattooed. And then uh, the 1 thing that you mentioned bar is the Google example, right? Because I think this kind of is a perfect segue into the you know nuances of data quality when it comes to generative AI you mentioned that survey at the beginning of 100% exactly 100% of data leaders are pressure or you know, Under Pressure to deliver a generative AI use cases, right? Um, that does not sound surprising at all. So, you know, when if you're a data leader, if you're in an organization trying to build a generative AI use case, what are the data quality considerations you need to have are they different from the general data quality considerations, you need to have what are the nuances that you need to have? Uh, like what are the new ones when it comes to the considerations of the inequality when it comes to uh strength of AI so bar. I'll let you, you know continue on that.
00:33:34
Barr Moses
Yeah, I mean, I think look if if I think about sort of the state of den of AI with Enterprises today, I you know, I mentioned from the survey 100% are under pressure by the way, 91% are actually building something. So we've almost all of us have succumb to the pressure for whatever reason. Um, and uh,
00:33:53
Barr Moses
I think when we say we're building with generative AI that can take very definition. So I'll give you an example just last week. I spoke to 1 1 Enterprise customer who told me we have the full entire sort of like Tech stack for generative AI built, you know with all investing class. We're we're fully ready to go we have no, you know use cases or we we don't really know we don't have anything that's like tied to business outcome that we can point to but the tech stack is ready like we're ready to go and then and then um, you know, another customer that said we have you know, 300 or so business use cases laid out. We have like some great ideas for how to drive business. We have nothing on the tech stack they were totally we you know, we don't even know where to get started.
00:34:20
Adel Nehme
Mhm.
00:34:37
Barr Moses
Um, and I think that represents a spectrum of where customers are at. Um,
00:34:38
Barr Moses
You know can be anywhere on 1 versus the other side or in the middle. I think there's more questions than answers at this point A lot of people are experimenting or sort of in the early days of building things in in Pilots. Some of it is also in production. Um, but I think early days I think by and large across all of these instances companies understand that they have to make sure that the data that's actually serving those llms um has to be accurate and here's why
00:34:55
Adel Nehme
Mhm.
00:35:06
Barr Moses
today everyone has access to the best models, you know, the the the models being built by 5,000 phds and a billion dollars in gpus we can all access them. There's no competitive Advantage for a company with them. Where does a competitive Advantage lie, it's actually with the proprietary data that you can bring that could be, you know via rag or fine tuning whatever method you choose, but it's your proprietary data that will help differentiate your generative AI product so that you can create personalized experience for your customer or so that you can automate your own business process.
00:35:23
Adel Nehme
Mhm.
00:35:38
Barr Moses
But without that proprietary data, there's not really a moat or sort of competitive advantage and so companies are realizing that they need to get their proprietary data, um in strong shape and so that means making sure that that is a high high quality data and so we are seeing um, you know, more and more companies thinking about how do we get ready? So that when the time comes and we actually have the tech stack and the business use case and everything and we can actually deliver on that we have
00:36:06
Barr Moses
Have the right the right data, um, uh, and we can actually use it.
00:36:10
Adel Nehme
That's great. Can I bring more and then George I'll let you react to that as well.
00:36:15
George Fraser
Um, I like to comment about the models, uh, you know, everyone has access to the models and the access for differentiation is is uh, what data you put into them. I I have actually heard Consultants advise companies that because everyone has to has access to the public models. There's the way you need to differentiate is by making your own model, which is insane advice. Like yeah that will differentiate you it will differentiate you because your model will be much worse than everyone.
00:36:45
George Fraser
But uh, it's so early days. It's hard to speculate about this. I mean I
00:36:50
George Fraser
all the AI stuff is so embryonic. It's very exciting because it's giving uh us the ability to do something with the unstructured tech data that we've been we've had we've had it for years. Um, but it's it's giving us the ability to interact with unstructured text in a meaningful but programmatic way, um what that turns into, um time will tell I don't know if rag is going to be the be all end all um, I question whether chat is even the right long term interface for a lot of these internal applications. Um, but I don't have like a great alternative on the tip of my tongue. So I just I think it's very early days and everyone should have their eyes and ears open.
00:36:58
Adel Nehme
Mhm.
00:37:01
Adel Nehme
Mhm.
00:37:22
Adel Nehme
Mhm.
00:37:32
Adel Nehme
Yeah, and for a couple of from a data call Quality data governance perspective. How does generative AI change the conversation?
00:37:38
Prukalpa Sankar
Yeah, I think.
00:37:41
Prukalpa Sankar
I think it's very early but a few patterns we're seeing I think across all our customers were seeing this pattern of people deploying.
00:37:47
Prukalpa Sankar
Small language models, right? Uh more than like they're like, which is where Rags fine tuning like some of this comes in. That's 1 pattern we're seeing
00:37:49
Adel Nehme
Mhm.
00:37:55
Prukalpa Sankar
and as the look at that, I think the 2 newest outside of just normal data quality, which is anything to do that we're seeing is 1 the importance of
00:38:02
Adel Nehme
Mhm.
00:38:07
Prukalpa Sankar
Business terms and semantic context. So for example, we are a customer who is an investment firm and you know, he was like, you know when someone searches in our like someone chats saying cam in our company Tam means total addressable Market not the 8 thing that it means in the internet. So 1 layer is just like, how do you like if you get an accurate output? What is the semantic context that's core to the company and how do we feed that? Exactly? And that's 1 layer that's becoming very important or more important than before.
00:38:10
Adel Nehme
Mhm.
00:38:21
Adel Nehme
Yeah.
00:38:31
Adel Nehme
Mhm.
00:38:39
Prukalpa Sankar
the second is we are seeing uh around this is a little bit more around governance, but also relates a little bit of trust which is how do I
00:38:47
Prukalpa Sankar
Depending on who's writing a question what data actually goes into that answer. So for example, if I'm deploying something for my HR team, it's probably okay if payroll data gets used in the answer. It's probably not. Okay, if it is across the rest of the company, um, I buy data from LinkedIn for which has like certain terms and conditions associated with it. I can only use it for this purpose not this purpose. And so as you build that scale democratization, uh the way I think about this you you alluded to this right like which is the goal post keeps changing. Actually. I think that's a good thing. The reason the goalpost is changing is because people are using data more
00:38:53
Adel Nehme
Mhm.
00:39:04
Adel Nehme
Mhm.
00:39:09
Adel Nehme
Mhm.
00:39:29
Prukalpa Sankar
And the more people use data the more they they need to trust it. The more there are issues. Like it's a it's actually a good goal post. And so if you actually play this out
00:39:37
Prukalpa Sankar
like there's more and more people who are going to maybe the the dream of like truly democratized data where everybody actually uses data daily and like, you know, like that's going to play out. But then how do you feed it with the the right people should only get the right context at the right time and a way that's safe and secure. Like how do you solve for that? Uh, those problems is proliferating and now need to be done in a very different way than before so,
00:39:56
Adel Nehme
Mhm.
00:39:60
George Fraser
I you know, I think those problems of permissions are easier than your getting at a couple but the reason people think these problems are hard is because they look at
00:40:12
Prukalpa Sankar
because
00:40:14
George Fraser
That the people who make the base models like openai and anthropic and Mr. All and all uh, and they're doing like web scraping so they have a whole data pipeline. That's like a scraping pipeline that is in in design that on the assumption that it is public data. And so everything has the same permissions domain.
00:40:32
George Fraser
In their relational databases have text columns. They've had them for a long time. They work great. Uh, but there's also going to be a forest of other tables that tell you all of the permissions metadata that you need to know in order to manage this problem. So I think you know, if if you if you're starting point is like a web scraping pipeline that looks like what the people who train the base models are using. Yes, the permissions problem look very hard. But if your starting point is a relational database that is structured similarly to the 1 you use for bi uh, this is the whole problem. You just need to you need to join all the appropriate things and recapitulate the permissions rules of the systems of record in the SQL queries and you're ready to go. It's not that it's like, um, so easy you're going to do it in a day, but my point is it's not really new this idea of I have a database I have a bunch of data in it. There are rules about who is allowed to see what like that if if you have a complete schema of the system that you're talking about. That is a very solvable problem using traditional techniques.
00:41:37
Adel Nehme
Mhm.
00:42:06
Adel Nehme
And it's that software that sorry for me. I look at the question is does that solve through something like rag, but I'll let you answer your question. Uh, yeah continue.
00:42:07
Prukalpa Sankar
Yeah.
00:42:08
Prukalpa Sankar
Good.
00:42:14
Prukalpa Sankar
question, so I mean I think the
00:42:17
Prukalpa Sankar
I I don't give my call is for like what's the new technology we need. I actually think that I mean we I think we actually spoke about this maybe like 9 months ago on maybe a different panel like and we were like, you know, maybe the technology the data and Ai and it's likely you want to look pretty similar to what the
00:42:34
Prukalpa Sankar
Like I don't think it's a technology here. I do think the Nuance of solving the like it does introduce a lot of new new answers around like uh, just because you're processing this at a speed and at a scale and at like like there's actually nuances to this.
00:42:48
Prukalpa Sankar
which need to be solved for um, if we we move towards that AI World, um, and then second there's a uh, there's also a human collaboration problem, which is what is the policy
00:43:00
Prukalpa Sankar
Uh, it's not it's not even a technology problem. It's like how do we collaborate to figure out what the right policies are for what The Right Use cases are and that's like that used to happen on like people like I've seen so many examples of people doing this like there's documents written and like published somewhere. Nobody ever uses them. Uh, and it was okay because it was like a few dashboards here and there which is just not going to be okay in the future. So, how do you solve for them? I think that's why it becomes more important.
00:43:23
Adel Nehme
Mhm.
00:43:27
Adel Nehme
How are you able to kind of uh, put a cost on a data quality issues. So couple I'll start with you.
00:44:03
Prukalpa Sankar
I feel like Barb might have a better answer to this 1 um, but I because I know you have a framework that you put out at some point, but
00:44:11
Prukalpa Sankar
I think the high level your
00:44:14
Prukalpa Sankar
first is almost accepting that everything in data across.
00:44:19
Prukalpa Sankar
Cannot directly be measured to business value because data itself is a support function inside an org. It's like bizops and management things like so like if an analyst produced a report which say like rco talks about this like he was like, I was hiring a bunch of sales reps in my in my team at the same time. Like I hired 1 analysts who found this 1 thing that we could optimize and we actually like made an extra million dollars through that 1 analysts. What's the ROI of that analysts thing? It's way more than that sales reps. Uh, uh, so how do you think well, it's just harder to do because it's 2 layers removed because data needs to drive strategy or execution both of those things together Drive business away, uh, and it's just hard to recover. So that's true for any data platform tooling across and I think first just accepting that I think is helpful and then second then okay, then what are the so if you can't get the outcome metric what's the output metric that you can get to it is the way I think about it. So we do need a notch star that we can.
00:44:24
Adel Nehme
Mhm.
00:44:34
Adel Nehme
Mhm.
00:44:58
Adel Nehme
Yeah.
00:45:13
Adel Nehme
Mhm.
00:45:18
Prukalpa Sankar
Progress towards because you know that this is important to get to the outcome and you know, if you're in a company that you need to convince people on that then I like I would question whether like data is actually really important to the company, uh, because like that's pretty straightforward like, you know, you should have good data to dive that. Like if you're convincing people on that like I think the first question to really have with whoever is asking you for a business case is is this really important uh for you because if it's not then like then that's okay. Let's have a conversation about it. And then I think what's the metric and I'll let talk about that because I know you have a good
00:45:47
Adel Nehme
Yeah.
00:45:49
Adel Nehme
Yeah, I I let bar and and with us on the framework.
00:45:53
Barr Moses
Sure. So, um the number 1 thing. It depends on I'll say it depends on who you're who you're talking to if you talk to Executives the number 1 Thing they'll tell you is I just sleep better at night, uh knowing that someone sort of is that that the data that's powering my business, you know, whatever. It is Data products dashboard Jen of AI call whatever you like. I just like better at night knowing that the data is accurate, which is very hard to measure it to per call this point. Um, if you talk to data engineer machine learning engineer, whatever it is, um, they often time will talk about how much time they spend.
00:46:27
Barr Moses
And are they spending on sort of cleaning up data or cleaning up, you know fire drills, um and you know are are actually are they sort of, you know, building new pipelines and and doing things um doing other things. So that's you know, kind of like various answers that you will get I will say in general there's sort of 3 things that we think about the first is um reputation in brand and Trust. So when your data is wrong again, like, you know, think about the Google example, I don't know if I'll Trust another Google search again after I saw the superglue example, um, the second is cost of Revenue.
00:46:46
Adel Nehme
Mhm.
00:46:59
Barr Moses
Um, and so oftentimes, you know, I gave the airline example, but there's real applic real implications, you know, 1 data issue can easily cost millions of dollars for an organization. Um, and then the third metric that you know, I mentioned is sort of Team efficiency or or team time, uh, your organizational time on that. Those are sort of the 3 high level metrics.
00:47:19
Adel Nehme
Okay, that is and I think this is a great time to end today's panel and then day 1 of radar. Uh, I want to say a huge. Thank you for kalpa bar George for joining us for such an insightful session. I truly truly appreciate everyone show them the love of the Emojis.
00:47:36
Adel Nehme
Below and I also say a huge. Thank you for everyone joining from across the world, you know people joining us from different time zones people even like 2 mm 3 mm watching this stuff. I really really appreciate it. So I want to say a huge huge. Thank you to all panelists today and to our speakers, um and to our audience and in the meantime to check out the LinkedIn group, keep connecting and see you tomorrow same time. Same place. I really appreciate everyone.
00:48:02
Prukalpa Sankar
Thank you.
podcast
Building Trust Through Data with Prukalpa Sankar, Co-Founder of Atlan
podcast
Creating Trust in Data with Data Observability
In this episode, Adel speaks with Barr Moses, CEO, and co-founder of Monte Carlo, on the importance of data quality and how data observability creates trust in data throughout
podcast
[Radar Recap] Scaling Data ROI: Driving Analytics Adoption Within Your Organization with Laura Gent Felker, Omar Khawaja and Tiffany Perkins-Munn
podcast
[Radar Recap] From Data Governance to Data Discoverability: Building Trust in Data Within Your Organization with Esther Munyi, Amy Grace, Stefaan Verhulst and Malarvizhi Veerappan
podcast
[AI and the Modern Data Stack] Adding AI to the Data Warehouse with Sridhar Ramaswamy, CEO at Snowflake
podcast
Data Science at McKinsey
Hugo speaks with Taras Gorishnyy, a Senior Analytics Manager at McKinsey and Head of Data Science at QuantumBlack, a McKinsey company, about what it takes to change organizations through data science.